Navigating Enterprise AI Deployment Challenges and Best Practices
The goal of this article is to shed light on the key challenges enterprises encounter during AI deployment and offer actionable best practices to overcome them.

Artificial Intelligence (AI) is no longer a futuristic concept; it has firmly embedded itself into the core strategies of leading enterprises. From predictive analytics to process automation, data and AI solutions are reshaping industries by enhancing efficiency, reducing costs, and uncovering new opportunities. However, while the potential of AI is boundless, deploying it successfully within an enterprise environment is fraught with challenges. According to a 2024 Gartner report, over 70% of AI projects fail to move beyond proof-of-concept stages. This alarming statistic underscores the complexities that enterprises face when integrating AI into their operations.
At its core, the promise of AI lies in its ability to transform data into actionable insights, automate repetitive tasks, and enable smarter decision-making. But achieving these outcomes requires navigating a landscape of technical, organizational, and ethical hurdles. Enterprises must contend with issues such as data silos, lack of talent, integration complexities, and regulatory compliance. Moreover, even the most robust AI systems can falter if organizational culture and change management strategies are not aligned.
The goal of this article is to shed light on the key challenges enterprises encounter during AI deployment and offer actionable best practices to overcome them. Whether you are a CTO strategizing your next AI initiative or a business leader looking to leverage AI for competitive advantage, this guide aims to equip you with the knowledge and tools to navigate the AI deployment journey effectively.
The challenges of enterprise AI deployment
Deploying AI at an enterprise level is a transformative yet complex journey. Organizations often face significant hurdles, from integrating AI into existing systems to managing the cultural shift it demands. Scaling AI solutions across departments requires not only robust infrastructure but also a clear strategy and alignment with business objectives.
1. Data readiness and quality
AI systems are only as good as the data they are trained on. Enterprises often grapple with data that is incomplete, unstructured, or scattered across disparate systems. Integrating and preparing this data for AI consumption requires significant effort and expertise. Moreover, ensuring that the data is unbiased and representative of real-world scenarios is critical to avoid skewed outcomes.
2. Talent scarcity
Despite the growing demand for AI capabilities, the supply of skilled professionals—including data scientists, machine learning engineers, and AI ethicists—remains limited. Compounding this issue is the need for cross-functional collaboration, where domain experts and technical teams must work in tandem to design and implement AI solutions.
3. Scalability
Many enterprises struggle to scale AI solutions beyond pilot projects. What works in a controlled proof-of-concept environment often encounters roadblocks when scaled across the organization. Challenges include integration with legacy systems, ensuring consistent performance, and managing computational costs.
4. Ethical and regulatory concerns
The adoption of AI raises critical ethical questions around transparency, fairness, and accountability. Additionally, enterprises must navigate a complex web of regulations—such as the EU’s General Data Protection Regulation (GDPR) or industry-specific compliance standards—to avoid legal pitfalls.
5. Change management
AI adoption often requires significant shifts in workflows, decision-making processes, and employee roles. Resistance to change from stakeholders, coupled with a lack of clear communication and training, can hinder adoption and limit the impact of AI initiatives.
Best practices for overcoming deployment challenges
Overcoming AI deployment challenges requires a strategic and structured approach. By focusing on best practices, such as fostering cross-functional collaboration, ensuring data quality, and aligning AI initiatives with business goals, organizations can pave the way for successful implementation. These practices not only address common obstacles but also maximize the value and impact of AI solutions.
1. Invest in data governance
Establishing robust data governance frameworks is a foundational step in AI deployment. This includes creating standardized data collection and storage processes, ensuring data security, and implementing mechanisms to monitor data quality. Additionally, fostering a data-driven culture—where employees at all levels understand the importance of data integrity—is crucial.
2. Build cross-functional teams
Successful AI projects require collaboration between technical experts and business stakeholders. By forming cross-functional teams, organizations can ensure that AI solutions are aligned with business objectives, are feasible to implement, and address real-world challenges effectively.
3. Start small, scale strategically
Rather than attempting to deploy AI across the organization at once, start with smaller, focused projects that deliver measurable results. These early wins can build momentum, secure stakeholder buy-in, and provide valuable insights for scaling AI initiatives.
4. Prioritize explainability and ethics
Ensure that AI models are interpretable and their decision-making processes are transparent. This not only builds trust among stakeholders but also mitigates risks associated with biased or unfair outcomes. Establishing a AI ethics committee to oversee deployments can further enhance accountability.
5. Emphasize change management
Proactively address resistance to change by involving employees in the AI adoption process. Provide training programs to upskill staff, highlight the benefits of AI, and communicate how it will enhance rather than replace their roles. Leadership buy-in and clear messaging are key to fostering a culture of AI acceptance.
6. Leverage cloud and automation tools
Cloud-based platforms and automation tools can significantly simplify the deployment and scaling of AI solutions. By leveraging these technologies, enterprises can reduce infrastructure costs, accelerate development timelines, and improve scalability.
7. Monitor and iterate
AI deployment is not a one-time effort; it requires continuous monitoring and refinement. Regularly evaluate the performance of AI systems, solicit feedback from users, and iterate on models to ensure they remain effective and aligned with business goals.
Conclusion
Deploying AI in an enterprise setting is a complex endeavor, but it is one that offers immense rewards for those who can navigate the challenges effectively. By addressing data readiness, fostering collaboration, prioritizing ethics, and embracing change management, enterprises can unlock the full potential of AI. Moreover, starting with small, strategic projects and leveraging modern tools can pave the way for scalable, sustainable AI adoption.
In the age of digital transformation, AI is not just a competitive advantage—it is a necessity. By adopting a thoughtful and structured approach to AI deployment, enterprises can position themselves at the forefront of innovation, driving value for their stakeholders and shaping the future of their industries.
What's Your Reaction?
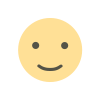
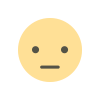
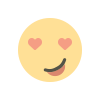
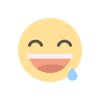
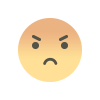
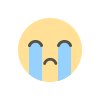
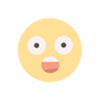