Kubernetes Autoscaling: Automating Resource Management for Your Containers
Managing resource allocation and scaling applications in Kubernetes can be a daunting task, especially when dealing with unpredictable workloads. One of the most powerful features of Kubernetes autoscaling, which enables your clusters to automatically adjust their resources based on real-time demand. This capability ensures that applications run efficiently, with minimal manual intervention, while avoiding underutilization or over-provisioning of resources.
At Kapstan, we help companies leverage Kubernetes' full potential by implementing autoscaling strategies that optimize performance and cost. In this article, we’ll dive deep into how Kubernetes autoscaling works, the types of autoscaling available, and how it can improve your infrastructure management.
What Is Kubernetes Autoscaling?
Kubernetes autoscaling is a feature that automatically adjusts the number of running containers (pods) and the resources available to them based on demand. This dynamic scaling helps to ensure high availability and resource efficiency without requiring manual intervention.
Kubernetes offers several types of autoscaling:
-
Horizontal Pod Autoscaling (HPA): This adjusts the number of pods based on observed CPU utilization or other custom metrics.
-
Vertical Pod Autoscaling (VPA): This changes the resource requests and limits for containers within a pod.
-
Cluster Autoscaling: This adjusts the number of nodes in your cluster based on resource demand and utilization.
Together, these autoscaling mechanisms enable Kubernetes clusters to automatically scale up or down to meet the demands of your workloads.
Horizontal Pod Autoscaling (HPA)
Horizontal Pod Autoscaling is one of the most common types of autoscaling in Kubernetes. It works by automatically increasing or decreasing the number of pods based on resource usage, typically CPU or memory utilization. HPA ensures that your application can handle traffic spikes without manual intervention, and conversely, it can scale down to save costs when demand is low.
For example, if you have a web application running in Kubernetes and the traffic increases, HPA will automatically create additional pods to handle the increased load. When the traffic drops, HPA will reduce the number of pods, preventing wasted resources.
Kubernetes Autoscaling in Action
When implementing Kubernetes autoscaling, HPA can be configured with specific thresholds. For instance, you might set HPA to scale the application when the CPU usage exceeds 80% or when a custom metric (e.g., request count) reaches a predefined limit.
This Kubernetes autoscaling ensures that applications remain responsive while optimizing resource allocation. With the right configuration, you can maintain high availability and minimize operational costs.
Vertical Pod Autoscaling (VPA)
While HPA focuses on adjusting the number of pods, Vertical Pod Autoscaling adjusts the resources allocated to a pod. This type of autoscaling is useful when your workloads require varying CPU or memory resources but don’t need more instances of a pod. VPA automatically adjusts the CPU and memory requests and limits for running pods to ensure they are allocated the right amount of resources based on usage patterns.
VPA is often used in scenarios where certain applications have fluctuating resource requirements but do not necessarily require horizontal scaling. For example, a large data processing job may require more CPU during peak processing hours, but the job can run efficiently with fewer resources when not executing.
Cluster Autoscaling
In addition to scaling pods and individual containers, Kubernetes can also scale the cluster itself. Cluster Autoscaling adjusts the number of nodes in the cluster based on the resources required by the running workloads. If your cluster is underutilized, it will scale down by removing nodes; conversely, if the demand for resources exceeds the current capacity, Kubernetes will automatically add new nodes to the cluster.
Cluster Autoscaling helps maintain optimal resource usage, ensuring that your infrastructure grows and shrinks dynamically as demand fluctuates. This can result in significant cost savings, as you’re only paying for the resources you need, when you need them.
Why Kubernetes Autoscaling Is Essential
Implementing Kubernetes autoscaling helps teams handle dynamic workloads efficiently. The benefits are clear:
-
Cost Efficiency: Autoscaling ensures that you only use the resources you need. When traffic is low, the system scales down to reduce costs.
-
High Availability: By scaling up during high-demand periods, Kubernetes helps ensure that applications remain available and responsive.
-
Performance Optimization: Autoscaling ensures that applications are properly resourced, preventing over-provisioning or under-provisioning of hardware resources.
-
Reduced Operational Overhead: With autoscaling, your team doesn’t need to manually manage scaling. Kubernetes automatically adjusts, allowing your team to focus on other tasks.
At Kapstan, we help our clients configure autoscaling for their Kubernetes clusters, ensuring that the scaling policies are optimized for both performance and cost-efficiency.
How to Implement Kubernetes Autoscaling
Implementing autoscaling in Kubernetes typically involves setting up the required metrics and configuring autoscaling policies. Here’s a high-level overview of the steps involved:
-
Install Metrics Server: The Metrics Server collects resource usage data (like CPU and memory) from your pods and nodes. This is required for HPA and VPA.
-
Configure HPA or VPA: Define the scaling rules based on your workload’s requirements. For HPA, set target metrics like CPU or memory usage.
-
Set Up Cluster Autoscaling: Install and configure the Cluster Autoscaler to dynamically add or remove nodes based on your cluster’s resource needs.
-
Monitor and Adjust: Continuously monitor the performance of your autoscaling setup and adjust the rules and thresholds as needed.
By following these steps and tailoring the configurations to your workloads, you can create a highly automated, cost-effective infrastructure with Kubernetes autoscaling.
Conclusion
Kubernetes autoscaling is a powerful tool that helps optimize resource management in your containers and clusters. By automatically adjusting the number of pods, their resource allocation, and even the nodes in your cluster, Kubernetes ensures that your applications are always available and running efficiently. Whether you’re scaling horizontally, vertically, or at the cluster level, autoscaling is an essential part of maintaining a robust and cost-effective cloud-native infrastructure.
At Kapstan, we guide businesses in setting up and fine-tuning their Kubernetes autoscaling configurations, ensuring that your systems are both scalable and optimized for performance. Ready to take your infrastructure to the next level? Contact us today, and let’s build a cloud-native solution that grows with your needs.
What's Your Reaction?
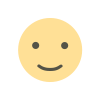
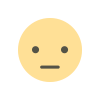
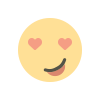
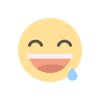
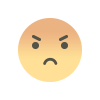
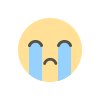
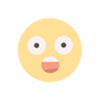